Cory Doctorow: What Kind of Bubble is AI?
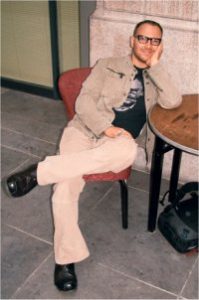
Of course AI is a bubble. It has all the hallmarks of a classic tech bubble. Pick up a rental car at SFO and drive in either direction on the 101 – north to San Francisco, south to Palo Alto – and every single billboard is advertising some kind of AI company. Every business plan has the word “AI” in it, even if the business itself has no AI in it. Even as two major, terrifying wars rage around the world, every newspaper has an above-the-fold AI headline and half the stories on Google News as I write this are about AI. I’ve had to make rule for my events: The first person to mention AI owes everyone else a drink.
It’s a bubble.
Tech bubbles come in two varieties: The ones that leave something behind, and the ones that leave nothing behind. Sometimes, it can be hard to guess what kind of bubble you’re living through until it pops and you find out the hard way.
When the dotcom bubble burst, it left a lot behind. Walking through San Francisco’s Mission District one day in 2001, I happened upon a startup founder who was standing on the sidewalk, selling off a fleet of factory-wrapped Steelcase Leap chairs ($50 each!) and a dozen racks of servers with as much of his customers’ data as I wanted ($250 per server or $1000 for a rack). Companies that were locked into sky-high commercial leases scrambled to sublet their spaces at bargain-basement prices. Craigslist was glutted with foosball tables and Razor scooters, and failed dotcom T-shirts were up for the taking, by the crateful.
But the most important residue after the bubble popped was the millions of young people who’d been lured into dropping out of university in order to take dotcom jobs where they got all-expenses paid crash courses in HTML, Perl, and Python. This army of technologists was unique in that they were drawn from all sorts of backgrounds – art-school dropouts, humanities dropouts, dropouts from earth science and bioscience programs and other disciplines that had historically been consumers of technology, not producers of it.
This created a weird and often wonderful dynamic in the Bay Area, a brief respite between the go-go days of Bubble 1.0 and Bubble 2.0, a time when the cost of living plummeted in the Bay Area, as did the cost of office space, as did the cost of servers. People started making technology because it served a need, or because it delighted them, or both. Technologists briefly operated without the goad of VCs’ growth-at-all-costs spurs.
The bubble was terrible. VCs and scammers scooped up billions from pension funds and other institutional investors and wasted it on obviously doomed startups. But after all that “irrational exuberance” burned away, the ashes proved a fertile ground for new growth.
Contrast that bubble with, say, cryptocurrency/NFTs, or the complex financial derivatives that led up to the 2008 financial crisis. These crises left behind very little reusable residue. The expensively retrained physicists whom the finance sector taught to generate wildly defective risk-hedging algorithms were not able to apply that knowledge to create successor algorithms that were useful. The fraud of the cryptocurrency bubble was far more pervasive than the fraud in the dotcom bubble, so much so that without the fraud, there’s almost nothing left. A few programmers were trained in Rust, a very secure programming language that is broadly applicable elsewhere. But otherwise, the residue from crypto is a lot of bad digital art and worse Austrian economics.
AI is a bubble, and it’s full of fraud, but that doesn’t automatically mean there’ll be nothing of value left behind when the bubble bursts. WorldCom was a gigantic fraud and it kicked off a fiber-optic bubble, but when WorldCom cratered, it left behind a lot of fiber that’s either in use today or waiting to be lit up. On balance, the world would have been better off without the WorldCom fraud, but at least something could be salvaged from the wreckage.
That’s unlike, say, the Enron scam or the Uber scam, both of which left the world worse off than they found it in every way. Uber burned $31 billion in investor cash, mostly from the Saudi royal family, to create the illusion of a viable business. Not only did that fraud end up screwing over the retail investors who made the Saudis and the other early investors a pile of money after the company’s IPO – but it also destroyed the legitimate taxi business and convinced cities all over the world to starve their transit systems of investment because Uber seemed so much cheaper. Uber continues to hemorrhage money, resorting to cheap accounting tricks to make it seem like they’re finally turning it around, even as they double the price of rides and halve driver pay (and still lose money on every ride). The market can remain irrational longer than any of us can stay solvent, but when Uber runs out of suckers, it will go the way of other pump-and-dumps like WeWork.
What kind of bubble is AI?
Like Uber, the massive investor subsidies for AI have produced a sugar high of temporarily satisfied users. Fooling around feeding prompts to an image generator or a large language model can be fun, and playful communities have sprung up around these subsidized, free-to-use tools (less savory communities have also come together to produce nonconsensual pornography, fraud materials, and hoaxes).
The largest of these models are incredibly expensive. They’re expensive to make, with billions spent acquiring training data, labelling it, and running it through massive computing arrays to turn it into models.
Even more important, these models are expensive to run. Even if a bankrupt AI company’s model and servers could be acquired for pennies on the dollar, even if the new owners could be shorn of any overhanging legal liability from looming copyright cases, even if the eye-watering salaries commanded by AI engineers collapsed, the electricity bill for each query – to power the servers and their chillers – would still make running these giant models very expensive.
Do the potential paying customers for these large models add up to enough money to keep the servers on? That’s the 13 trillion dollar question, and the answer is the difference between WorldCom and Enron, or dotcoms and cryptocurrency.
Though I don’t have a certain answer to this question, I am skeptical. AI decision support is potentially valuable to practitioners. Accountants might value an AI tool’s ability to draft a tax return. Radiologists might value the AI’s guess about whether an X-ray suggests a cancerous mass. But with AIs’ tendency to “hallucinate” and confabulate, there’s an increasing recognition that these AI judgments require a “human in the loop” to carefully review their judgments.
In other words, an AI-supported radiologist should spend exactly the same amount of time considering your X-ray, and then see if the AI agrees with their judgment, and, if not, they should take a closer look. AI should make radiology more expensive, in order to make it more accurate.
But that’s not the AI business model. AI pitchmen are explicit on this score: The purpose of AI, the source of its value, is its capacity to increase productivity, which is to say, it should allow workers to do more, which will allow their bosses to fire some of them, or get each one to do more work in the same time, or both. The entire investor case for AI is “companies will buy our products so they can do more with less.” It’s not “business customers will buy our products so their products will cost more to make, but will be of higher quality.”
AI companies are implicitly betting that their customers will buy AI for highly consequential automation, fire workers, and cause physical, mental and economic harm to their own customers as a result, somehow escaping liability for these harms. Early indicators are that this bet won’t pay off. Cruise, the “self-driving car” startup that was just forced to pull its cars off the streets of San Francisco, pays 1.5 staffers to supervise every car on the road. In other words, their AI replaces a single low-waged driver with 1.5 more expensive remote supervisors – and their cars still kill people.
If Cruise is a bellwether for the future of the AI regulatory environment, then the pool of AI applications shrinks to a puddle. There just aren’t that many customers for a product that makes their own high-stakes projects better, but more expensive. There are many low-stakes applications – say, selling kids access to a cheap subscription that generates pictures of their RPG characters in action – but they don’t pay much. The universe of low-stakes, high-dollar applications for AI is so small that I can’t think of anything that belongs in it.
Add up all the money that users with low-stakes/fault-tolerant applications are willing to pay; combine it with all the money that risk-tolerant, high-stakes users are willing to spend; add in all the money that high-stakes users who are willing to make their products more expensive in order to keep them running are willing to spend. If that all sums up to less than it takes to keep the servers running, to acquire, clean and label new data, and to process it into new models, then that’s it for the commercial Big AI sector.
Just take one step back and look at the hype through this lens. All the big, exciting uses for AI are either low-dollar (helping kids cheat on their homework, generating stock art for bottom-feeding publications) or high-stakes and fault-intolerant (self-driving cars, radiology, hiring, etc.).
Every bubble pops eventually. When this one goes, what will be left behind?
Well, there will be little models – Hugging Face, Llama, etc – that run on commodity hardware. The people who are learning to “prompt engineer” these “toy models” have gotten far more out of them than even their makers imagined possible. They will continue to eke out new marginal gains from these little models, possibly enough to satisfy most of those low-stakes, low-dollar applications. But these little models were spun out of big models, and without stupid bubble money and/or a viable business case, those big models won’t survive the bubble and be available to make more capable little models.
There are some promising avenues, like “federated learning,” that hypothetically combine a lot of commodity consumer hardware to replicate some of the features of those big, capital-intensive models from the bubble’s beneficiaries. It may be that – as with the interregnum after the dotcom bust – AI practitioners will use their all-expenses-paid education in PyTorch and TensorFlow (AI’s answer to Perl and Python) to push the limits on federated learning and small-scale AI models to new places, driven by playfulness, scientific curiosity, and a desire to solve real problems.
There will also be a lot more people who understand statistical analysis at scale and how to wrangle large amounts of data. There will be a lot of people who know PyTorch and TensorFlow, too – both of these are “open source” projects, but are effectively controlled by Meta and Google, respectively. Perhaps they’ll be wrestled away from their corporate owners, forked and made more broadly applicable, after those corporate behemoths move on from their money-losing Big AI bets.
Our policymakers are putting a lot of energy into thinking about what they’ll do if the AI bubble doesn’t pop – wrangling about “AI ethics” and “AI safety.” But – as with all the previous tech bubbles – very few people are talking about what we’ll be able to salvage when the bubble is over.
Cory Doctorow is the author of Walkaway, Little Brother, and Information Doesn’t Want to Be Free (among many others); he is the co-owner of Boing Boing, a special consultant to the Electronic Frontier Foundation, a visiting professor of Computer Science at the Open University and an MIT Media Lab Research Affiliate.
All opinions expressed by commentators are solely their own and do not reflect the opinions of Locus.
This article and more like it in the December and January 2023 issue of Locus.
While you are here, please take a moment to support Locus with a one-time or recurring donation. We rely on reader donations to keep the magazine and site going, and would like to keep the site paywall free, but WE NEED YOUR FINANCIAL SUPPORT to continue quality coverage of the science fiction and fantasy field.
©Locus Magazine. Copyrighted material may not be republished without permission of LSFF.
Good article, but I’m not sure that I 100% agree. For radiology, the BEST review would indeed be the more expensive mix of human plus AI (and some places are now doing this and charging a surcharge for use of AI). However there is at least some studies that suggest AI is not better at reading mammograms than a human radiologist. So the best, most expensive option is human + AI, the 2nd best and cheapest is AI, and the worst and 2nd most expensive is human (only). If the business model for AI + human doesn’t fly, I can see AI only working fine.
On automated vehicles, Cruise is a good example of what can go wrong, but Waymo is having significantly better results. I think the jury is still out on that one.
On LLM, I agree with you. I do ask two questions (and it’s an ask, I’m ignorant in this area): 1) are the newly emerging small models fully derivatives from the massive models, or might that continue even if the massive models are a bubble? 2) There are a lot of applications where training is done on massive data sets and hardware, but the resulting trained models can then run at far lower cost, on MUCH smaller machines. Is this also a possible continued path if LLM’s are a bubble that burst?
Curious that author does not dwell more on the fact, that use cases like GitHub copilot will actually make any programming faster and easier, also lower the entry threshold for new workforce which will be most likely very positive.
As a programmer with some experience under my belt – copilot doesn’t make “real” (outside demos) programming much faster, it gives maybe a few percent gain. A lot of that could also be gained just by improving libraries and frameworks used to reduce repetitive and/or boilerplate code.
Utility for biolerplate and glue is EXTREMELY useful, since those are the demoralizing activities humans dont want to concern ourselves with, that take significant time.
Its also a substitute for reading a full manual when you only want to use a singular feature of something for a specific purpose.
But we already have _much_ better techniques for getting rid of boilerplate code: better languages, better libraries, refactorings, and so on.
Keep in mind that a lot more time is spent _reading_ code than writing it; you can’t modify existing code unless you can read it and be sure what it does and what the effects of your changes will be. So generating more boilerplate code might fix a few problems in the very short term (over a few weeks, or often even just days), but creates a larger problem (often referred to as “technical debt”) over the long term.
I have tried using ChatGPT to give me hints on how to write code, but I’ve inevitably found that, while it’s been useful for giving me (almost invariably incorrect) rough draughts in languages I’m less familiar with, it works only because I’m already a good programmer in many other languages, and so I can fairly easily edit that draught into useful and correct code.
Copilot is usally a nuisance that slows down experienced programmers.
I’m not finding Copilot to be that useful. It makes lots of wrong suggestions, too.
Totally agree.
AI will probably prove to be a bad solution (at least for now) for a lot of the domains where it’s being attempted, for the simple reason that a lot of the output is bullshit.
But there is a lot of money in products and services where “it’s bullshit” isn’t a show-stopper. Mostly entertainment, but there is a lot of money in that. I recently participated in a D&D campaign where the DM made very liberal use of AI, both for text/running encounters, and for image generation. The AI clearly wasn’t yet good enough to do the whole thing on it’s own, but it was much better and more useful than expected, and clearly reduced the workload on the DM a lot, while also greatly improving immersion.
What I’m saying is that in a couple of years the experience of videogaming will change a lot, and this will probably produce enough revenue to keep the bubble going, at least for a while.
They want, so bad, for it to replace the GM too but that is such a complicated job that I suspect they might find self driving cars easier. Those GM emulators are fun for a bit but rapidly get repetitive or just lose the thread and they are terrible at knitting together an overarching narrative out of pieces and chaos like a good human GM can do.
Cory Doctorow’s piece on AI as a bubble provides a nuanced perspective on the current AI landscape.
He argues convincingly that AI is in a bubble phase, akin to previous tech bubbles, where hype and investment overshadow practical utility and sustainable business models. Doctorow draws parallels with the dotcom bubble, where despite the burst, valuable skills and infrastructure remained.
He points out the distinct lack of tangible, beneficial remnants from other bubbles, like cryptocurrency/NFTs and the 2008 financial crisis derivatives.
Validating Doctorow’s Opinions:
1. AI Overhype: Doctorow’s assertion that AI is overhyped and likened to a bubble seems valid. The ubiquity of AI in business plans, news headlines, and advertising, regardless of their actual AI integration, mirrors the classic signs of a technology bubble.
2. Historical Bubble Analysis: His analysis of different bubbles, like the dotcom and cryptocurrency bubbles, and their aftermaths is insightful. The contrast between bubbles that leave behind valuable assets, skills, or infrastructure (dotcom) and those that don’t (cryptocurrency/NFTs) is a valuable framework to assess the potential impact of the current AI bubble.
3. Skepticism About AI’s Business Model: Doctorow’s skepticism about the AI business model’s sustainability and its potential to deliver value is grounded. He highlights the mismatch between the promise of increased productivity and the real need for human oversight in AI applications, which raises questions about the long-term viability of these technologies.
Countering Doctorow’s Opinions:
1. Underestimating AI’s Potential: While Doctorow rightly points out the overhype and potential for fraud in AI, he might underestimate the technology’s transformative potential. AI’s capacity for data analysis, pattern recognition, and decision support can revolutionize fields like healthcare, finance, and more, beyond just being a productivity tool.
2. Broad Generalization of AI: Doctorow’s argument, at times, seems to broadly generalize AI technologies, not fully acknowledging the diversity within the field. Not all AI applications are equivalent; some, like machine learning models in healthcare and environmental sciences, show substantial promise and utility.
3. Overlooking Positive Use Cases: His focus on the negative aspects, while crucial, might overshadow the positive, real-world applications of AI that are already making an impact. For instance, AI’s role in medical diagnostics, climate modeling, and even creative arts, though in their nascent stages, demonstrate a constructive trajectory.
In conclusion, Doctorow’s opinions on the AI bubble are largely well-founded, especially his critique of the overhyped nature of AI and the parallels with historical tech bubbles. However, his perspective might benefit from a more nuanced acknowledgment of the positive, transformative potential of AI in various sectors.
Thanks, ChatGPT, that was a good summary of what I just read.
Dang, you got me.
Even in the countering points it suggests no low stakes high value use cases and only doubles down on the point of medical use cases, which the author already covered. This barren absence of use cases that are more than making a complete amateur, 1 rudimentary subject in any knowledge area better, is exactly the point of what is making this a bubble.
Exactly. It was a regurgitation of the same talking points we’ve all been hearing for months now… with the word “nuanced” thrown in. I guess ChatGPT interpreted that Cory was coming across a little too direct? lol
It was obvious, as are many comments.
AI requires editing to remove the bland exposition of all points of view.
Be great for writing a paper on the morality of intersex dysphoria treatment.
Did you submit this to your English 101 class?
I lived through the 2000 tech bubble burst.
I noticed that it started about 97-98.
At times it seemed that if you stuck “on the internet” with anything on a napkin in a bar you could get financed.
I have a feeling that AI will go that way where lots of businesses go bust and lots of investors lose their shirts but the world will be forever changed but not the way people pre-bubble thought.
If you could not copyright anything generated by AI what would that do to those who want to create and own content.
There would not be much point in using AI to create that blockbuster movie script if you could not claim you own it.
This is a nice comparison to previous bubbles. However, it leaves out the thing this bubble was driven by, other bubbles less so: a vast accumulating body of academic research in neural network architectures, deep learning theory, and domain-specific applications. There is a LOT that will be left when the bubble pops. In some ways this can be compared to the development of web based technology that accompanied the dotcom boom, but it is much more substantial. Consider for example AlphaFold, the solution to a 50 year fundamental problem in protein bioinformatics. That’s a whole lot more than just some CSS and JavaScript frameworks and the Model-View-Controller paradigm.
Yes, and AlphaFold is not based on generative AI. AI was everywhere before November 2022, and it’s not going anywhere. GenAI and LLMs are the bubble.
Interesting article, but very static analysis in a very dynamic field. The hardware cost issues will most likely be address by some combination of Moore’s Law and Application Specific Integrated Circuits (ASICs), so that is a short term hardware issue.
The software also has a lot of room for optimization. Particularly with zero weights. It may also turn out that the saying about Neural Nets, that they are the technology of the future and always will be, is true. Who creates a system that mucking large with no instrumentation? That’s eng101.
As for accuracy, they need to start training with a None Of The Above (NOTA) category. We used to use it all the time in the days before NNs when simple feature vectors and convolution filters were used. Once a calc falls below a confidence level, it gets flagged. So with the radio example, the AI picks off all the easy ones. The ones that are obviously clean or cancerous. The hard ones get flagged for a second look. Using multiple AIs and voting is also an effective approach. But what ever they do they must instrument the mucking things. It has to be able to explain why it made this choice. IDing every spot on the X-ray and labeling it, is a start.
The current products are absurdly bad. Ask it anything it doesn’t already know, and it looses it’s mind. Even things it should know, are not immune. Again, Eng101, before you do anything with it, ask it questions you already know the answers to. Don’t even trust the fact check. I’ve seen cases where the model was right and the fact check got it wrong. You just can’t make this spit up.
As always, just my $0.02 worth. It may all just be an AI hallucination.
Doctorow is being pretty reductive here – LLMs (specifically the transformer algo) are being used for so many interesting things I can’t imagine we’re going to run out of giant data sets ripe for mining for meaningful discoveries any time soon. That’s the real application of transformer AI – making sense of enormous amounts of data.
For instance – protein folding – Deepmind’s Alphafold basically short-cutted the human race in that area by about 20 years. Same thing for material science – they used the same technique to discover a couple of hundred thousand different material compositions that we’re working with now to find the useful ones.
Then of course there’s DNA interaction, all of the medical records in the world, deep space scan research, etc…. there’s sooooo much data out there just waiting to be devoured by an LLM there’s got to be a crapload of money on the table.
I’m not sure AI is a bubble, but mainly because I’m hopeful that by learning from AI and machine learning we can learn about human intelligence. New research of AI discovering a new class of antibiotics (https://www.scientificamerican.com/article/new-class-of-antibiotics-discovered-using-ai/) or helping to control nuclear fusion (https://www.wired.com/story/deepmind-ai-nuclear-fusion/) to the point where ignition has now been achieved multiple times makes me think AI or at least some version of it is here to stay.
I don’t think the whole article is correctly set. There’s a lot of talk about AI but it’s misleading since we should talk about Generative AI which has gotten mainstream this year, while AI as a general technology has already in use for decades. Talking about GenAI, there’s certainly a lot of hype around it but it is a great assistive tool to enhance several fields of human work, even if we are still in early stages. A lot of improvement could and should be made but it is a promising direction and I don’t see the reason to criticize too early, but I agree on reasoning and discuss about it.
Whoever says ‘bubble’ first after this comment buys the comment section drinks.
Good analysis. I was deeply immersed in the fiber-optic portion of the dot.com bubble, and the fundamental problem there were that the technology got a decade or more ahead of the demand for bandwidth. That, in turn, was powered by Worldcom’s claim that internet traffic was doubling every three months — which may have been true for one company for quarter around 1995, but was not sustained. No carriers were willing to release data on actual transmission growth because they all considered it proprietary, so the hustlers made up numbers, and the market wanted to believe.
I remember worrying about the actually fiber capacity and demand back around 1999, and thinking thinking this irrational exuberance could not sustain itself, but the dot-com crash didn’t spread to fiber until late in 2000. What happened was that nobody looked down. It was like the laws of cartoon physics; Wyle E Coyote’s legs kept churning after he ran beyond the edge of the cliff and the law of gravity did not take hold until he looked down. And when the market did look down and see how far they were above the ground, the market dropped like a rock, and big tech stocks dropped to pennies on the dollar.
I think the large scale AI providers trying to corner the AI market is definitely a bubble and the large scale AI can solve it fantasy is currently a fantasy. However even they are making an immediate and fundamental changes. All of our developers are absolutely dependent on AI for bug checking and finding incremental solutions much faster as they provided the creativity to what they are building.
As someone who specifically works adjacent to Radiologists working in AI and Deep Learning they have been working on imaging specific AI since long before the current bubble. It is about carefully training rigorous models on controlled data sets to improve accuracy and efficiency. They presume multiple layers of QA and project better overall outcomes. Dealing with PHI absolutely limits how and what kind of third parties are involved. Having dedicated experts working on refining specific closed AI systems is required to avoid poisoning.
My point being that AI is more than just the current bubble and those more focused incremental change areas will have long term impact. The current AI hype and fraud you discuss will also have negative long term impacts but that is no different than any other corporate fraud bubble.
The answer I suspect is that there will be a limited capacity regardless of cost effectiveness so only the most profitable will even be supportable. National Security seems to be the most likely beneficiary with the NSA, FBI and DoD using it for things like threat analysis, early warnings, missile targeting, radar enhancement, etc. Places where the scope can be defined sufficiently that accuracy and reliability are substantial. The other place I would guess is agriculture as rising chemical costs drive farmers to need tools to identify soil conditions, plant health, weed growth and water needs in real time. Another set of data than can be relatively simply built and operated.
Replacing US workers writ large is going to take AGI and that’s not even conceptually related to these LLM programs.
Over 20 years ago, I was told that the AI embedded in speech recognition software would replace human court stenographers and closed captioners “any day now.”
I’m still waiting.
This one example proves technology improvement never happens.
Sarcasm don’t? Because we may not have those particular applications doesn’t mean that AI hasn’t improved voice recognition by multiple orders of magnitude, leading to new applications, including smart speakers, automated translation that is good enough for many use cases, and more.
That’s not quite the logical leap I would make, but you do you.
Court reporters being replaced by technology just happens to be my personal litmus test for whether or not I start taking recent claims about AI seriously.
Keep in mind that court reporters in many venues make 6-figure salaries.
There’s a huge difference between “technology never improves” and “AI is currently over hyped.” The first is demonstrably false, including for AI. The latter is hard to disagree with, and I certainly wouldn’t.
AI’s abilities has grown by multiple orders of magnitude and is now routinely used in many new applications AND, at the same time, it is greatly over hyped.
Are we investing more in AI than its potential worth? I would agree that many of today’s firms will go bust, especially those who think that an AI version of Petsmart will dominate the world.
But I wonder: NVIDIA became a trillion-dollar firm, but it wasn’t entirely hype-based. They have been “eating their own dogfood” by using stacks of their hardware to speed up the design and validation of subsequent versions of their chippery. A particularly notable example is the development of CuLitho, which accelerated computational lithography forty-fold. I can cite many other instances where AI has already led to vast improvements in productivity.
Today’s chatty LLMs are rife with problems, but they’re not the Omega of the process, but the leading edge. Many other approaches are designed to be truthful and transparent and useful; from my survey of arxiv preprints, a great deal of substantial improvements are in the wind.
The author conflates overinvestment bubbles with other types of crises / bubbles such as accounting fraud (Enron), or debt crisis (2008 / 2009). This apples and pears comparison makes that part of the analysis invalid.
Overinvestment bubbles usually leave behind the assets that were overinvested in. The first / most famous case was the US railways in the 1800’s. The fiber optic overinvestment mentioned is another great example. One could argue that all government infrastructure programs are in some ways like that, not justified by economic calculations within the normal time horizons but accretive over the long term nevertheless.
So in that way of course something will be left behind.
A useful part of the analysis is the 2×2 on monetizability (!) vs tolerance to errors. I agree the venn diagram is narrow. The supporters of the AI case usually point to the rate of improvement and to the fact that we barely started exploring the use cases.
AI seems to work for well bounded problems and less well for open problems.
If AI drops the cost of coding software by an order of magnitude it will have already paid for itself.
You people imagining that LLMs will make “coding” easier or faster (laughably “drop the cost of coding software by an order of magnitude”!) probably don’t have much experience in software development. Writing the code is a very small part. Putting it together, testing it, maintaining it, enhancing it and above all finding and fixing bugs takes far longer. Right now LLMs are best at creating bugs – what they write always looks plausible but usually isn’t 100% right. LLMs don’t just take the best and most expert-reviewed code as their learning, they take ALL the code. Garbage in, garbage out. I hope my competitors rely on “AI” code, I really do.
I’ve been playing around with ChatGPT to aid in writing and debugging software and I agree 100% with your assessment of the current state of the art.
The question is can and will this change? Personally I think it’s an open question.
6 months ago artists were mocking midjourney for not being able to draw hands.
the state of the art ones draw hands quite well.
Totally agree. If someone thinks developing a software means just Writing code then its a pretty naive understand of what software development is. These are “managers” who think its actually the non technical stuff like “business ” that matters when it somes to product development 🙂 .. in reality success of a product depends entirely on maintainability in terms of technical side i.e cost..
And ability to write maintable software is an art not a science. It will be very hard to capture that unless we are taking about ai that can truly replace a human brain.. i.e. give it customer requirements and it outputs the product.
Personally, I expect at least benefits in communicating to and from automated systems.
But, setting everything else aside, let’s remember the energy cost of generative AI. The next few years are critical for our energy transition and the planet needs the help from all of us. I think we need to hold cloud services accountable to transition to fully renewable energy, with an extra emphasis in not negatively impacting other services and industries transitions to renewables on our (shared in most cases) grid.
The way programmers are talking about this right now reminds me of various earlier fads that ended up leaving massive amounts of cleanup and administrative work for decades in their wake. I have absolutely no programming skills myself so I can’t comment further on that.
I think both sides of this argument have clear, ulterior motives, but basically only Doctorow, his comrades in arms, and maybe one or two of the pro “AI” set are being honest about what they are. Doctorow’s is, while technically no less ulterior, in the end really much better served by honesty. if he gets this wrong, for example, it will be much more lucrative for him to market his work, as having been improved by learning from the failure than if he simply asserts to have always been right. That kind of stuff might fly in certain political circles online, but they aren’t the ones he has clear incentives to work. while other ulterior motives on display in the comment thread, like desiring prolonged control of a budget, actually would be quite threatened from having been demonstrated wrong. It is hardly a coincidence that these are exactly the people claiming that the bubble tech in question is actually intelligent in some meaningful way such that calling it AI isn’t a lie.
Now we are talking political linguistics, which is more of my wheelhouse. while the article does also use the buzzword AI, the truth of that name is really outside the scope of the piece. This is less appreciably the case for certain arguments in the comments, which speak of it as currently measurable and significant intelligence we should not hasten to distrust. That only raises more questions for me.
Case in point: after a relatively painless transcription with apple speech to text, the compulsory “AI” rewrite forced me to intervene in every sentence multiple times to fix errors added after I had visually checked the transcription and still missed several. While I don’t get Perl, I have never made grammatical or stylistic errors like those in my life. I am a disabled person currently being coerced to manually enter text, at slight but real cost to my mental and physical health- if it were literally any more difficult I wouldn’t have bothered- to clean up after “AI” from this little upstart called APPLE COMPUTERS.
And this is supposed to get BETTER after the bubble pops?
The point of AI in this article like most of what is being written misses the boat completely. Where AI will make a difference is incredible fast, automated scientific discovery. Google is already developing hundreds of new materials using AI, accelerating protein folding, and advancing fusion. Apparently Deepmind has an algorithm to stabilize fusion. Microsoft just made a bet with Helion energy with a power purchasing agreement for fusion energy eight years from now. There is a prediction, that in the next 10 years, there will be 50 to 100 years of scientific progress in automated research done by AI. A lot of what is in the news misses the boat completely. The bubble will pop and we will find ourselves with a whole lot of new scientific breakthroughs in energy and materials.
This reminds me of Michael Kinsley’s confident prediction in the early 1980s that the AIDS kerfuffle would blow over when people got bored with it. But diseases don’t go away due to boredom.
But with medical development and safe sex, AIDS did weaken as a menace over the years. But AI won’t weaken, it will just get stronger.
Many people seem to be missing the point.
It’s not whether these algorithms work or not, or are even fit to purpose in some cases. That’s not really what defines a bubble. Bubbles are built on useful stuff, like the 2008 housing bubble. Houses are good, far better than living in a tent. The bubble was on people throwing more money at the houses and loans paying for those houses than those things would ever be worth.
These kinds of algorithms have been used for many years already for all kinds of useful stuff. Scanning handwritten text into usable text in a computer. Or speech recognition. Good stuff that takes horrible drudge work from humans.
A bubble is when the current investment fad is tossing money into something where there’s no clear return on investment, and instead of backing off when concerns are expressed, doubling down and throwing more money at it. And when too much money is flying around the scammers and grifters start showing up to skim a little (or a lot) of that money for themselves.
For many people, to admit the initial investment, or the obvious scam, they’ve just thrown millions of dollars into might be an error is more difficult than throwing more money at it and ignoring, or attacking, any criticism of the thing.
What Cory is saying here, and what many other critics of so called AI are saying, is the cost of running LLMs is far in excess of what anyone will pay for them, and there’s a limit to their capabilities that means you still need experienced professionals to do complex and difficult jobs. That even if Waymo figured out how to get their self-driving cars to be monitored by .75 humans and don’t kill anyone they’d still be losing money and go out of business eventually; the investors will lose their shirts while the VC’s and founders and scammers will come out of it all with bags full of money.
I can’t tell if the article is saying there will be useful stuff left over when the bubble pops or not. Personaly, I think the AI mania has already caused too much destruction. The greenhouse gases barfed out to build and power the server farms alone is too high a cost.
The technology will improve enormously and prices will come down correspondingly. AI is guaranteed to make some people fortunes. Of course you’re correct that it will also probably ruin many more.
Bubbles are not always built on useful stuff. Consider the 17th century Dutch tulip mania. A tulip bulb, while not entirely useless, was not a new invention, and was not adding any significant utility to society that it had not already been adding for centuries before the bubble.
Gen-AI has a strong tendency to hallucinate… traditional (non-gen) AI does not.
“There will be a lot of people who know PyTorch and TensorFlow, too – both of these are “open source” projects, but are effectively controlled by Meta and Google, respectively.”
PyTorch is now governed by the PyTorch Foundation, an independent organization within the Linux Foundation, so Meta does not control it anymore.